

Thanks weasal – i would also like to see the same drive thru style bottle o as well!
#Semeval 2015 task3 no cd
basic xe qr 101 000 automatic transmission, power window no cd player xe qr 111 000 cd player m cruise control, alloy wheel, power window… etc go for the first two, extra frozen and do n’t forget the caviar check mate …īasic ones fs, fully loaded ones will cost much moreĢ009 models are on offer. Good to clean house piping russian standard, zubrovka, movscoscaya not passing 100 qr. Anyone know why Pathfinder is so cheap?ĭid the prices come down or is it a good offer price? I thought Pathfinder is more expensive than Xttera. Saw an ad in today’s GT… some offer for Nissan Vehicles. Hey guys just wondering, what is the cost of a bottle of vodka in doha ? I dont mean from a hotel, but from the single bottle shop that is set up there. The final answer is labeled as ‘potentially useful’ because while it does not provide a relevant answer to the question, it contains a relevant advice for the user.Įxample of two questions and four of their answers from the SemEval 2015 dataset The third answer is ‘bad’ because it is completely irrelevant to the question. These two answers are both semantically relevant to the question, even though they might be inaccurate or completely wrong. In these examples, for each question, the first and the second answers are labeled as ‘good’ as both try to answer the question. Most of the questions and answers in these forums are often lengthy, informal, and contain abbreviations and grammatical mistakes. 1 1 As shown in this table, in CQA, each question has at least three parts: (i) question category, which is the category that the question belongs to (ii) question subject, which summarizes the question, and (iii) question body, which describes the question in details, and might contain useless or noisy parts as well. Table 1 shows two examples of questions, each with four answers, taken from the SemEval 2015 dataset. It should be noted that a good answer is an answer semantically relevant to the question, not necessarily the correct answer. As defined in SemEval 2015, in this task, the goal is to classify the answers given a question into three categories: (i) good, which are the answers that address the question well (ii) potentially useful to the user (e.g., because they can help educate him/her on the subject) (iii) bad or useless. In this paper, we address the task of answer selection. Thus, it is essential to automatically identify the best answers for each question. It means one has to go through all possible answers for assessing them, which is exhausting and time-consuming. Unfortunately, there is often no evaluation of the given answers in how much they are related to the question. In these forums, anyone can ask any question, and a question is answered by one or more members. Many applications such as sentiment analysis, recommender systems, relation extraction, and question answering integrate the information in KGs by linking the entities mentioned in the text to entities in the KGs.Ĭommunity question answering (CQA) forums, such as Stack Overflow and Yahoo! Answer provide new opportunities for users to share knowledge. They consist of entities and relationships among them. Knowledge graphs (KGs), such as DBpedia and BabelNet, are multi-relational graphs. The experimental results on three widely used datasets demonstrate that our proposed method is effective and outperforms the existing baselines significantly.

Moreover, the model uses variational autoencoders (VAE) in a multi-task learning process with a classifier to produce class-specific representations for answers. It also uses the question category for producing context-aware representations for questions and answers.

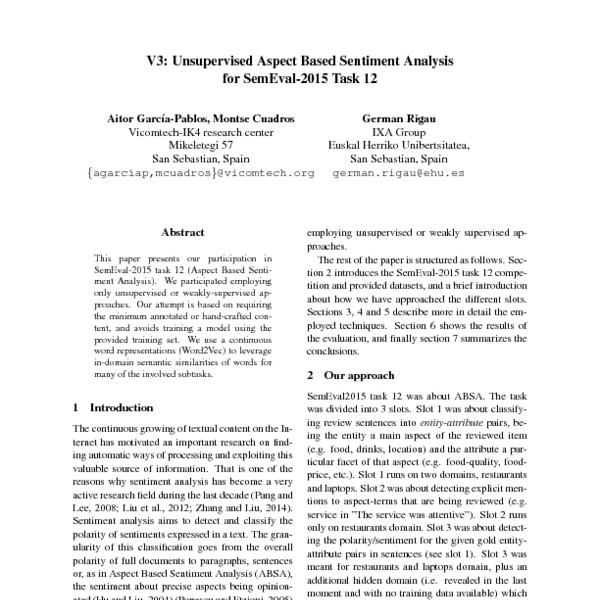
We also learn a latent-variable model for learning the representations of the question and answer, jointly optimizing generative and discriminative objectives. In this paper, we propose a novel answer selection method in CQA by using the knowledge embedded in KGs. Despite the obvious usefulness of commonsense and factual information in the KGs, to the best of our knowledge, KGs have been rarely integrated into the task of answer selection in community question answering (CQA). With the increasing popularity of knowledge graph (KG), many applications such as sentiment analysis, trend prediction, and question answering use KG for better performance.
